Artificial intelligence-based Modeling Mechanisms for Material Analysis and Discovery
Keywords:
Material analysis,Modeling mechanisms, AI simulations,Material analysisAbstract
Artificial intelligence-based materials application is one of the hot topics in the field of materials science. Materials are widely used in the space industry, cutting tools, thermal and electrical insulators, and refractory materials. The conventional experiments and statistical approaches usually require more resources and time. Thus, the need for Artificial intelligence applications in the simulation and exploration of novel materials is increasing. Recently, AI has been applied to materials for improving efficiency and prediction accuracy; however, there are many limitations due to the lack of benchmark datasets, advanced pre-processing mechanisms, prediction modelling mechanisms, and simulation tools in the materials literature. Thus it is challenging to identify optimal learning models, including algorithm selection, the architecture of models, data processing, and simulation mechanisms. In this paper, we attempt to review experimental and computational data-based AI mechanisms. Furthermore, the current research status is analyzed for using artificial intelligence techniques in material simulation tools and discovering new materials. Finally, we present research issues of AI-based application realization in materials science.
References
Iqbal, N., & Kim, D. H. (2022). Iot task management mechanism based on predictive optimization for efficient
energy consumption in smart residential buildings. Energy and Buildings, 257, 111762.
Jamil, F., & Kim, D. (2021). An ensemble of prediction and learning mechanism for improving accuracy of
anomaly detection in network intrusion environments. Sustainability, 13(18), 10057.
Imran, Imran, et al. "Using Machine Learning Algorithms for Housing Price Prediction: The Case of Islamabad
Housing Data." Soft Computing and Machine Intelligence 1.1 (2021): 11-23.
Imran, M., Zaman, U., Imtiaz, J., Fayaz, M., & Gwak, J. (2021). Comprehensive survey of iot, machine learning,
and blockchain for health care applications: A topical assessment for pandemic preparedness, challenges, and
solutions. Electronics, 10(20), 2501.
Zaman, U., Mehmood, F., Iqbal, N., Kim, J., & Ibrahim, M. (2022). Towards Secure and Intelligent Internet of
Health Things: A Survey of Enabling Technologies and Applications. Electronics, 11(12), 1893.
Qayyum, F., Kim, D. H., Bong, S. J., Chi, S. Y., & Choi, Y. H. (2022). A Survey of Datasets, Pre-processing,
Modeling Mechanisms, and Simulation Tools Based on AI for Material Analysis and Discovery. Materials,
(4), 1428.
Olson, G.B., 2000. Designing a new material world. Science, 288(5468), pp.993-998.
Sitek, W., Trzaska, J. and Dobrzański, L.A., 2006. An artificial intelligence approach in designing new materials.
Journal of Achievements in Materials and Manufacturing Engineering, 17(1-2).
Ramprasad, R., Batra, R., Pilania, G., Mannodi-Kanakkithodi, A. and Kim, C., 2017. Machine learning in
materials informatics: recent applications and prospects. npj Computational Materials, 3(1), pp.1-13.
Iqbal, N., Khan, A. N., Rizwan, A., Qayyum, F., Malik, S., Ahmad, R., & Kim, D. H. (2022). Enhanced timeconstraint aware tasks scheduling mechanism based on predictive optimization for efficient load balancing in
smart manufacturing. Journal of Manufacturing Systems, 64, 19-39.
Virshup AM, Contreras-García J, Wipf P, Yang W, Beratan DN. Stochastic voyages into uncharted chemical
space produce a representative library of all possible drug-like compounds. J Am Chem Soc 2013;135(19):7296–
link1
T. Zhou, Z. Song, and K. Sundmacher, 'Big Data Creates New Opportunities for Materials Research: A Review
on Methods and Applications of ML for Materials Design', Engineering, vol. 5, no. 6, pp. 1017–1026, Dec. 2019,
doi: 10.1016/j.eng.2019.02.011.
Jain A, Ong SP, Hautier G, Chen W, Richards WD, Dacek S, et al. Commentary: the materials project: a
materials genome approach to accelerating materials innovation. APL Mater 2013;1(1):011002. link1
Michalski RS, Carbonell JG, Mitchell TM, editors. ML: an AI approach. Berlin: Springer-Verlag; 2013. link1
Agrawal A, Deshpande PD, Cecen A, Basavarsu GP, Choudhary AN, Kalidindi SR. Exploration of data
science techniques to predict fatigue strength of steel from composition and processing parameters. Integr
Mater Manuf Innovation 2014;3:8.
Karak SK, Chatterjee S, Bandopadhyay S. Mathematical modeling of the physical and mechanical properties
of nano-Y2O3 dispersed ferritic alloys using evolutionary algorithm-based neural network. Powder Technol
;274:217–26. link1
Pilania G, Mannodi-Kanakkithodi A, Uberuaga BP, Ramprasad R, Gubernatis JE, Lookman T. ML bandgaps
of double perovskites. Sci Rep 2016;6:19375. link1
Jinnouchi R, Asahi R. Predicting catalytic activity of nanoparticles by a DFTaided machine-learning algorithm.
J Phys Chem Lett 2017;8(17):4279–83. link1
Zhou T, Jhamb S, Liang X, Sundmacher K, Gani R. Prediction of acid dissociation constants of organic
compounds using group contribution methods. Chem Eng Sci 2018;183:95–105. link1
Aghaji MZ, Fernandez M, Boyd PG, Daff TD, Woo TK. Quantitative structure– property relationship models
for recognizing metal organic frameworks (MOFs) with high CO2 working capacity and CO2/CH4 selectivity
for methane purification. Eur J Inorg Chem 2016;2016(27):4505–11. link1
Sharma V, Wang C, Lorenzini RG, Ma R, Zhu Q, Sinkovits DW, et al. Rational design of all organic polymer
dielectrics. Nat Commun 2014;5:4845. link1
Jha, D., Ward, L., Paul, A., Liao, W.K., Choudhary, A., Wolverton, C. and Agrawal, A., 2018. Elemnet: Deep
learning the chemistry of materials from only elemental composition. Scientific reports, 8(1), pp.1-13.
Ward, L., Dunn, A., Faghaninia, A., Zimmermann, N. E., Bajaj, S., Wang, Q., ... & Jain, A. (2018). Matminer: An
open-source toolkit for materials data mining. Computational Materials Science, 152, 60-69.
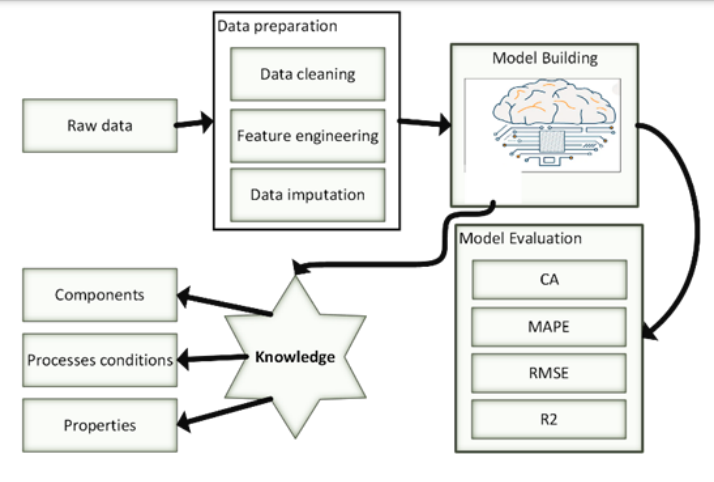
Downloads
Published
How to Cite
Issue
Section
License
Copyright (c) 2022 Journal of Intelligent Pervasive and Soft Computing

This work is licensed under a Creative Commons Attribution 4.0 International License.
Articles are licensed under an open access Creative Commons CC BY 4.0 license, meaning that anyone may download and read the paper for free.